Usage and example of github actions matrix
Environment and Prerequisite
- Github Actions
Background
- I needed to execute CI/CD with the same tasks but by changing only a few values, so I applied it.
- Detailed usage and examples can be found at https://docs.github.com/actions/writing-workflows/choosing-what-your-workflow-does/using-a-matrix-for-your-jobs.
Usage
- According to the document, you can use it as shown below.
jobs.<job_id>.strategy.matrix
is where you define the variables and values for the matrix.- In the example below, the values corresponding to
version
are[10, 12, 14]
and the same applies toos
.
jobs:
example_matrix:
strategy:
matrix:
version: [10, 12, 14]
os: [ubuntu-latest, windows-latest]
Example
If you want to run Jekyll builds on multiple OS, you can write it as shown below. This is a workflow example actually used in this blog.
Code
- This include other steps but just look on
strategy
injobs
’sbuild
. - Define os on statement
os: [ubuntu-22.04, ubuntu-20.04, macos-latest]
and use it onruns-on: ${{ matrix.os }}
. - The documentation states that if
jobs.<job_id>.strategy.fail-fast
is set totrue
or its expression evaluates totrue
, GitHub will cancel all in-progress and queued jobs in the matrix if any job in the matrix fails. To allow remaining jobs to continue even if one job fails, I’ve set this tofalse
.
name: Jekyll Build CI
on:
push:
branches: [ main ]
pull_request:
branches: [ main ]
workflow_dispatch:
inputs:
ruby-version:
required: true
type: string
default: "3.2.2"
jobs:
build:
name: jekyll build
strategy:
matrix:
os: [ubuntu-22.04, ubuntu-20.04, macos-latest]
fail-fast: false
runs-on: ${{ matrix.os }}
steps:
- name: Checkout Repository
uses: actions/checkout@v4
- name: Setup Ruby
uses: ruby/setup-ruby@v1
with:
ruby-version: ${{ github.event.inputs.ruby-version }}
- name: Install Bundler
run: |
gem install bundler
- name: Install Dependencies
run: |
bundle install
- name: Build Jekyll Site
run: |
bundle exec jekyll build
Result
- To make it easier to understand, I’m attaching an image that shows the results of the execution.
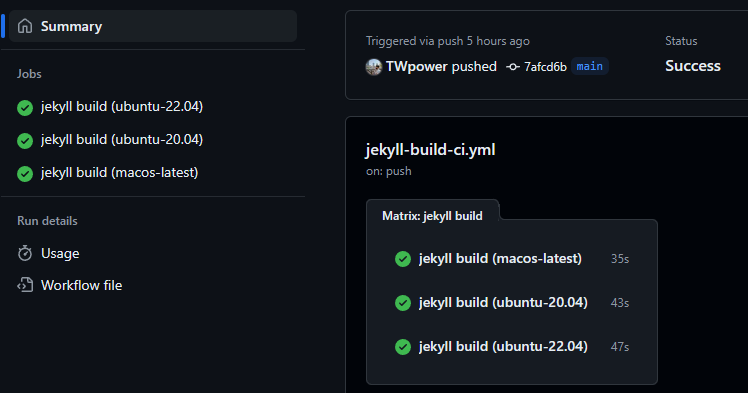